Surviving the Valley of Death
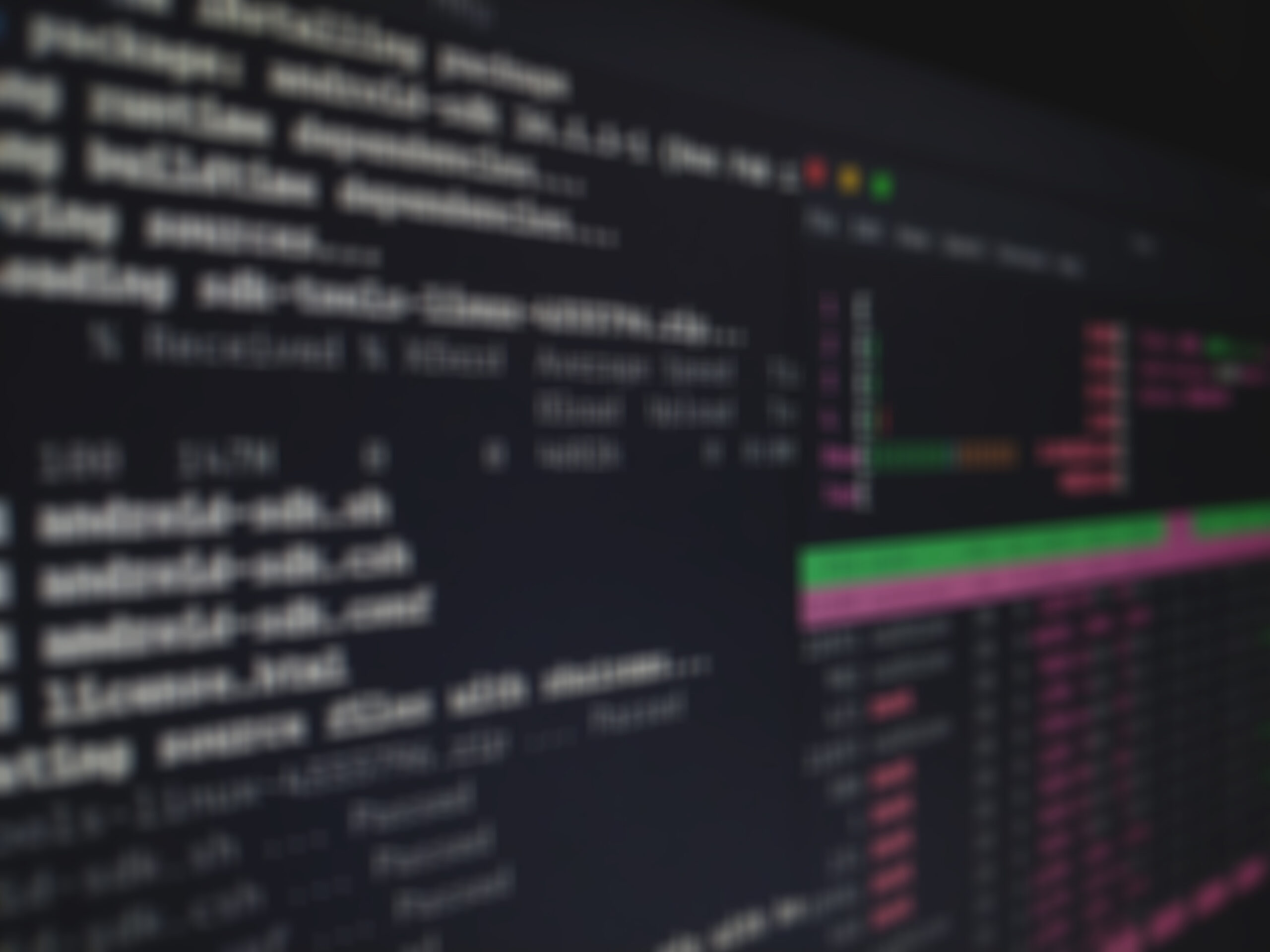
Search the web for “technology transition” and “valley of death” and you will run headfirst into an ongoing concern with the process of commercializing basic research (here’s one such report A Valley of Death in the Innovation Sequence: An Economic Investigation). As this report (and many other sources) characterize it, the Valley of Death in the innovation process occurs due to a “dearth of sources of funding for technology projects that no longer count as basic research but are not yet far enough along to form the basis for a business plan.” The result is that innovative technologies often go to the Valley to die a lingering death due to lack of bridge funding in the transition from idea to practice.
Over the years, various programs and initiatives have been created to address this challenge; for example, the excellent US Government SBIR/STTR program has been specifically designed to support the technology transition process. While this and other initiatives are certainly making a difference, in my opinion the Valley is growing wider and deeper with each passing year. Governments, in a worldwide struggle in which R&D is viewed as a competitive advantage, are funding ever more research, and a flood of money is arriving from philanthropists and non-profits (for example, in medical research) to push our basic scientific understanding in a diverse variety of fields including medicine, biology, materials, energy, computing systems, and many more. However, the gap remains because we are too focused on the discoveries and not the practical application of knowledge, which is where the impacts are really made. Many philanthropists are much more interested in discovering the cure for cancer than developing systems to deploy existing knowledge. Yet this is precisely where positive differences in the welfare of people are made most often.
My take is that the balance between the so-called three pillars of science — experiment, theory, and computation — is dramatically shifting in favor of much more computation; yet we have not manifested the corresponding processes to support the transition of computational discoveries nor maintain valuable resources (including software, data, publications and related services–see Data-Driven Science: A New Paradigm?). Many of today’s research projects do not recognize the scale to which data and science is driving innovation, and do not fully appreciate the need for skilled computational scientists, and evolved software and data processes to make the discoveries real.
In my experience, some in the scientific community view computation as a second-class tool to confirm theories, augment experiments, or process data; have a primitive understanding of software and the computational process; and do not recognize that the future of science is computation as the driving force behind discovery. As a result, there is an imbalance in funding and in the way research programs are organized. In many labs there is just enough research money to get the job done (e.g., publish a paper), but the behind-the-scenes software and data situation is a mess, meaning that the potential to transition the software to practice is negatively and significantly impacted. Thus, widening occurs as we push the “knowledge” side of the Valley away from the “practice” side.
Here’s a typical example I often see. When visiting a prestigious academic research group, a non-profit research organization, a commercial research center or even a national laboratory, I am often impressed and amazed at the work going on, but find myself completely aghast at the software processes (if any), data management practices, and even publication process. In many cases, source code is not held in a repository (every student has their own slightly-modified version); data, which costs millions of dollars to acquire, is placed on portable hard drives and stashed in filing cabinets; and complex computational processes are documented without regard to the principles of Open Access. Moreover, unreasonable IP barriers often rear their ugly head as code is viewed as proprietary or built on top of closed systems. All these and more make the technology transition process that much harder.
What to do about this? Start with openness, build a community, engage proficient software developers, and remove barriers to collaboration. Engage with companies like Kitware who have the smarts to engage in computational science, yet with the passion to build maintainable, reusable, and extensible software systems, processes and repositories. Hire more computational scientists and software people for the lab and treat them as first class citizens of the research team. Balance funding portfolios to recognize the increasing importance of computation in the innovation process. My belief is that by taking these steps to reduce the depth and breadth of the Valley, scientists will move their knowledge to practice much faster, make impacts much sooner, and manifest the practical applications of knowledge to receive accolades and future funding.