Brain extraction from normal and pathological images: A joint PCA/Image-Reconstruction approach
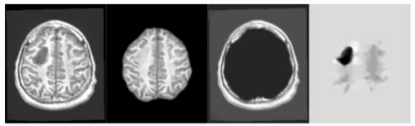
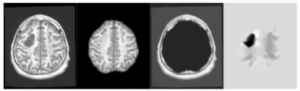
We have enjoyed many years of productive collaboration with Dr. Marc Niethammer (UNC), Dr. Roland Kwitt (U of Salzburg, Austria), and Dr. John Van Horn (USC): creating innovative algorithms, publishing them, and releasing their code as open-source software.
One of the problems that this team has been tackling is traumatic brain injury (TBI) assessment. In particular, we are developing methods that help register MRI scans taken after a TBI with standard anatomic atlases, so that we can more accurately localize the regions and extent of damage. The difficulty is that when major trauma has been sustained, it can be very difficult to map regions from the injured patient’s brain to a healthy atlas: tissues may have been drastically distorted, displaced, or destroyed. We have been pursuing a variety of methods to address this difficult problem [Neithammer 2011, Liu 2015]. Recently we have generalized some of that work to the broader task of delineating the boundary of the brain in medical scans, including scans containing large pathologies / injuries. Such “brain extraction” is a common step in many automated brain image analysis algorithms, including TBI analysis methods. That work is featured in the following journal article that was authored by one of Marc’s students and that appeared in the April issue of NeuroImage:
Xu Han, Roland Kwitt, Stephen Aylward, Spyridon Bakas, Bjoern Menze, Alexander Asturias, Paul Vespa, John Van Horn, Marc Niethammer, “Brain extraction from normal and pathological images: A joint PCA/Image-Reconstruction approach“, NeuroImage
Volume 176, 1 August 2018, Pages 431–445
This publication details the algorithms and includes comparisons with other methods for brain extraction. This work was funded, in part, by an NIH/NINDS grant (2R44NS081792), as well as several other NIH and NSF grants. As with most of this team’s grant-funded collaborations, the algorithms featured in this publication are freely available for academic and commercial use. See https://github.com/uncbiag/pstrip.
Congratulations Han!
— Stephen Aylward
Senior Director of Strategic Initiatives
Kitware, Inc.