SPIE Defense + Commercial Sensing 2024
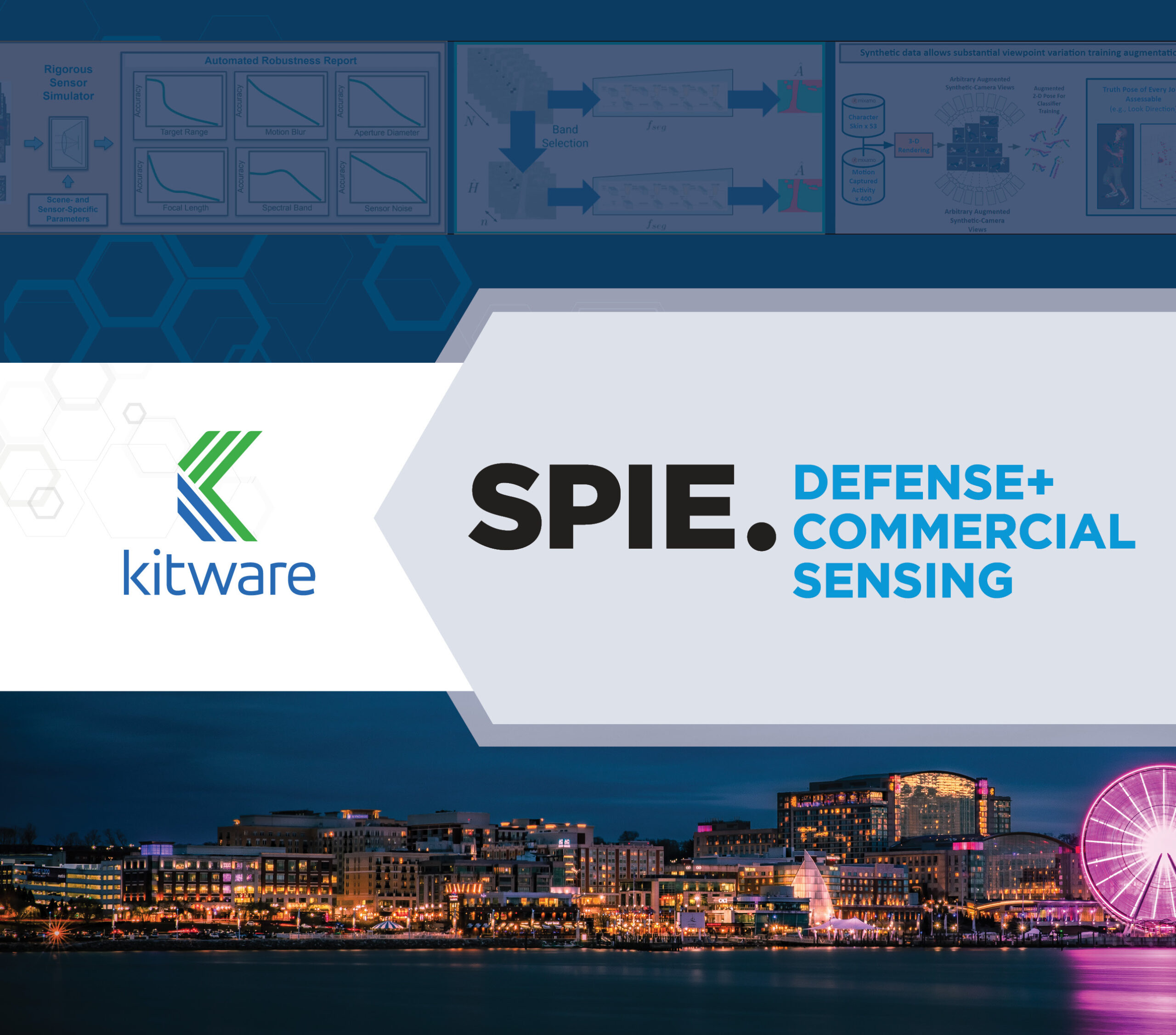
April 21-24, 2024 at the National Harbor in National Harbor, Maryland
The International Society for Optics and Photonics (SPIE) Defense + Commercial Sensing 2024 Conference brings together researchers and engineers to share the latest advancements in sensors, infrared, laser systems, spectral imaging, radar, lidar, autonomous systems, and other findings within the sensing community related to defense.
With more than two centuries of combined experience across Kitware in computer vision R&D for federal agencies, our highly experienced and credentialed experts can help you solve the most challenging AI and computer vision problems in space, from above, on the ground, and even underwater. With multiple invited talks and papers at this year’s gathering, Kitware is honored to be the most recognized and credentialed provider of advanced computer vision research across the DoD and IC, among companies focused on federal business. To learn more about our capabilities and how they can help you solve difficult research problems, schedule a meeting with our team.
Papers and Presentations
Improving visual AI models with synthetic, hybrid, and perturbed training data
Presenter: Anthony Hoogs, Ph.D.
Monday, April 22 8:30-9:10 AM ET | Potomac 6
Program Listing
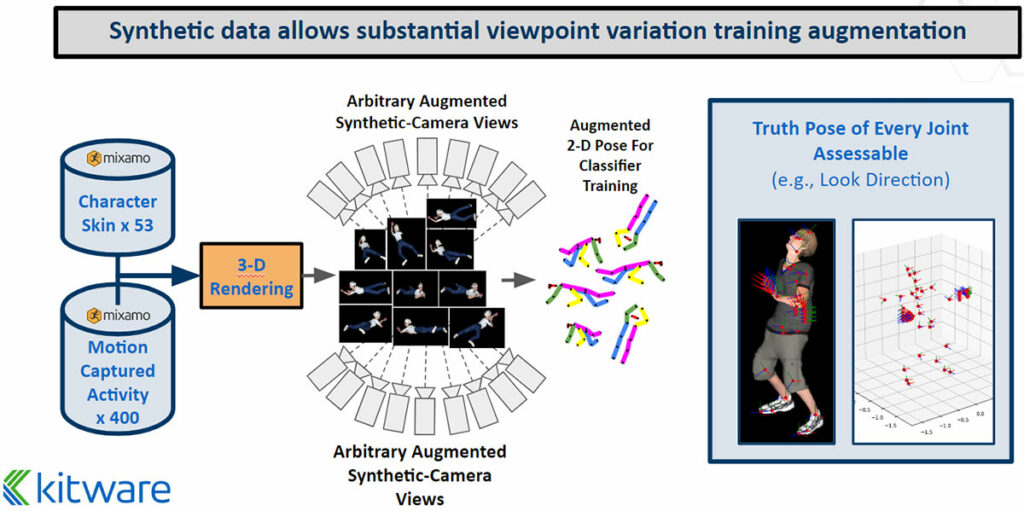
The use of generated data for training visual AI models has been increasing rapidly in recent years as the quality of AI-generated imagery has drastically improved. Since the beginning of the deep learning revolution about 10 years ago, deep learning methods have also relied on data augmentation to expand the effective size and diversity of training datasets without collecting or generating additional images. In both cases, however, the realism of the generated data is difficult to assess. Many studies have shown that generated and augmented data improve accuracy on real data, but when real test data has a significant domain shift from the training data, it can be difficult to predict whether data generation and augmentation will help to improve robustness. This talk will cover recent advances in realistic data augmentation, for applications where a target domain is known but has little test or training data. Under the CDAO JATIC program, Kitware is developing the Natural Robustness Toolkit (NRTK), an open-source toolkit for generating realistic image augmentations and perturbations that correspond to specified sensor and scene parameters. NRTK enables the significant expansion of test and training datasets to both previously unseen scene conditions and the realistic emulation of new imaging sensors that have different optical properties. Our results demonstrate that NRTK dataset augmentation is more effective than typical methods based on random pixel-level perturbations and AI-generated images.
End-to-end machine learning for co-optimized sensing and automated target recognition
Presenter: Scott McCloskey, Ph.D.
Tuesday, April 23 10:30-11:10 AM ET | National Harbor 5
Program Listing
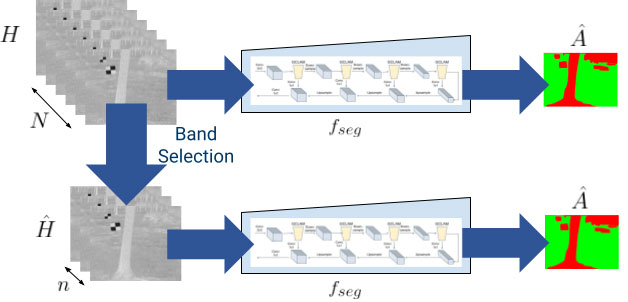
Many sensors produce data that rarely, if ever, is viewed by a human, and yet sensors are often designed to maximize subjective image quality. For sensors whose data is intended for embedded exploitation, maximizing the subjective image quality to a human will generally decrease the performance of downstream exploitation. In recent years, computational imaging researchers have developed end-to-end learning methods that co-optimize the sensing hardware with downstream exploitation via end-to-end machine learning. This talk will describe two such approaches at Kitware. First, we use an end-to-end ML approach to design a multispectral sensor that’s optimized for scene segmentation. In the second approach, we optimize post-capture super-resolution in order to improve the performance of airplane detection in overhead imagery.
Toward quantifying the real-versus-synthetic imagery training data ‘reality gap’: analysis and practical applications
Authors: Colin N. Reinhardt, Anthony Hoogs, Ph.D., Rusty Blue, Ph.D.
Thursday, April 25 8:30- 9 AM | Potomac 6
Program Listing
In the last few years, the methods used for the reconstruction of 3D geometry from imagery have undergone an AI revolution. These new methods are now being adapted to remote sensing applications as well. This training session will review recent developments in the computer vision and AI research communities as they pertain to 3D reconstruction from overhead imagery. We will provide a high-level summary of how traditional multi-view 3D reconstruction works, review the basics of neural networks, and then discuss how these fields have come together by reviewing and demystifying the recent research. In particular, we will discuss methods such as Neral Radiance Fields (NeRF) and Neural Implicit Surfaces for multiview 3D modeling. We will describe modifications and enhancements needed to adapt these methods from ground-level imagery to aerial and satellite imagery. We will also review recent work in using AI to estimate height maps from a single overhead image.
NRTK: an open source natural robustness toolkit for the evaluation of computer vision models
Presenter: Brian Hu, Ph.D.
Monday, April 22 3-3:20 PM ET | National Harbor 8
Program Listing
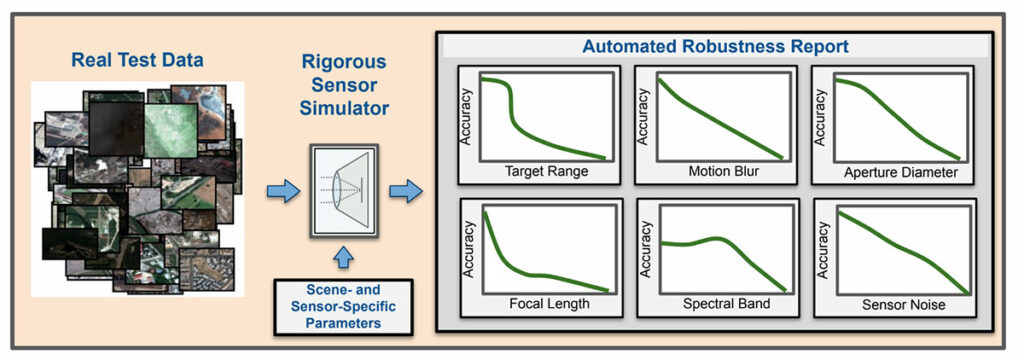
We introduce the open source Natural Robustness Toolkit (NRTK), a platform for validated scene- and sensor-specific perturbations for evaluating the robustness of computer vision models. We demonstrate how the NRTK can be used to evaluate computer vision models on aerial imagery. It can be paired with visualization tools to enable a more detailed understanding of datasets and their perturbations. We envision the NRTK to be a critical component of the test and evaluation suite for computer vision models.
About Kitware’s Computer Vision Team
Kitware is a leader in AI/ML and computer vision. We use AI/ML to dramatically improve object detection and tracking, object recognition, scene understanding, and content-based retrieval. Our technical areas of focus include:
- Multimodal large language models
- Deep learning
- Dataset collection and annotation
- Interactive do-it-yourself AI
- Object detection, recognition, and tracking
- Explainable and ethical AI
- Cyber-physical systems
- Complex activity, event, and threat detection
- 3D reconstruction, point clouds, and odometry
- Disinformation detection
- Super-resolution and enhancement
- Computational imaging
- Semantic segmentation
- We also continuously explore and participate in other research and development areas for our customers as needed.
Kitware’s Computer Vision Team recognizes the value of leveraging our advanced computer vision and deep learning capabilities to support academia, industry, and the DoD and intelligence communities. We work with various government agencies, such as the Defense Advanced Research Project Agency (DARPA), Air Force Research Laboratory (AFRL), the Office of Naval Research (ONR), Intelligence Advanced Research Projects Activity (IARPA), and the U.S. Air Force. We also partner with many academic institutions, such as the University of California at Berkeley, Columbia, Notre Dame, the University of Pennsylvania, Rutgers, the University of Maryland at College Park, and the University of Illinois, on government contracts.
To learn more about Kitware’s computer vision work, visit our expertise page or contact our team. We look forward to engaging with the SPIE community and sharing information about Kitware’s ongoing research and development in AI/ML and computer vision as it relates to the latest in imaging.