DARPA SemaFor: Defending Against AI-Generated Content
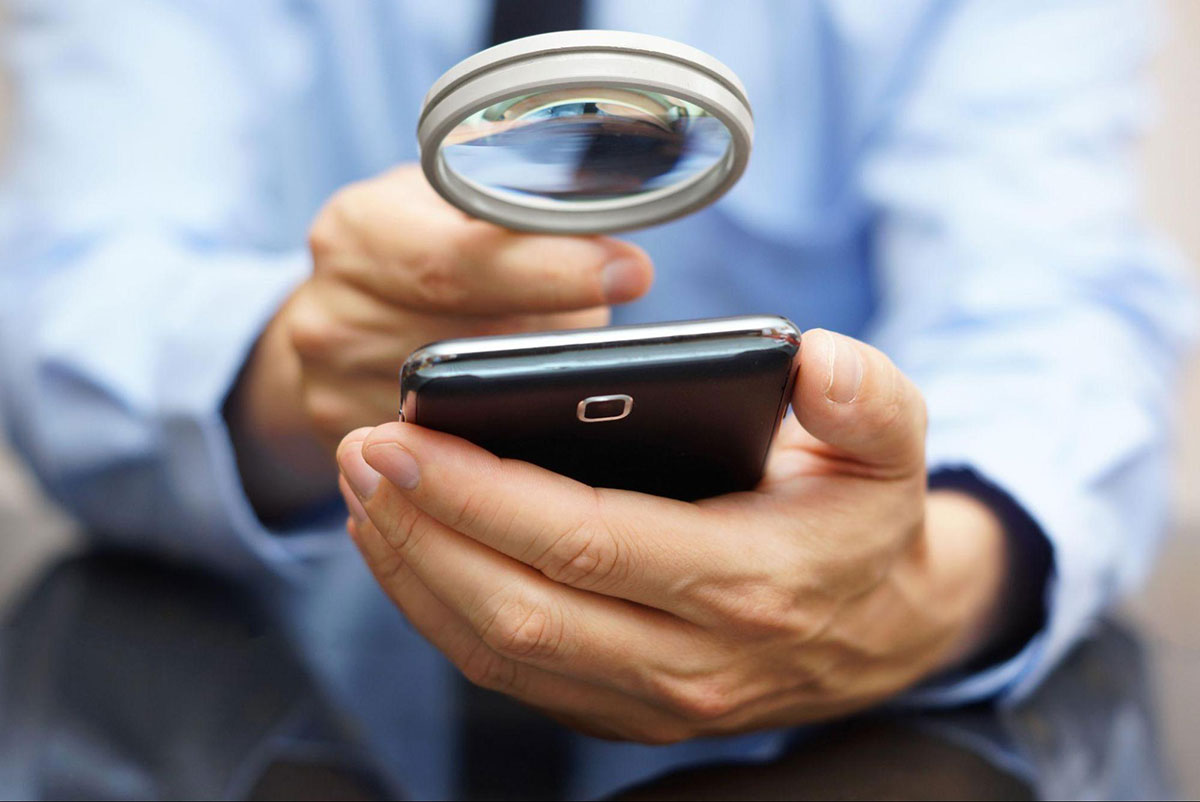
The Growing Challenge of AI-Generated Content
Generative AI has rapidly evolved, making it easier than ever to create synthetic text, images, and audio. Tools like OpenAI’s ChatGPT and Microsoft Copilot have put powerful AI capabilities into the hands of millions. While these advancements bring innovation, they also introduce serious risks.
Manipulated videos and synthetic audio can now make individuals appear to say or do anything, leading to real-world consequences such as eroding public trust, spreading propaganda, and changing digital content. As these threats grow, so does the need for advanced tools to detect, analyze, and counteract manipulated media.
To address this challenge, the Defense Advanced Research Projects Agency (DARPA) launched the Media Forensics (MediFor) and Semantic Forensics (SemaFor) programs. Our team at Kitware has been a leading force in these efforts, developing cutting-edge tools to identify and analyze AI-generated media across multiple formats, including text, images, audio, and video.
Fighting Media Manipulation: A Three-Pronged Approach
Our research focuses on three core areas: Detection, Attribution, and Characterization—each playing a vital role in countering the misuse of AI-generated media.
- Detection: Identifying synthetic content
Detection serves as the first line of defense against AI-generated media, helping to differentiate between authentic and synthetic content across text, images, audio, and video. Our research focuses on developing robust detection models that can adapt to evolving generative AI techniques.- Text: Our detection methods analyze linguistic structures and stylometric features, training models to differentiate between AI- and human-generated content. We use adversarial learning techniques that adapt to evolving generative models, ensuring robust detection even as text generators improve.
- Images: We developed classifiers that leverage frequency-based features and deep learning models to detect AI-generated images, even after post-processing edits like compression, resizing, and filtering. Our system also generates synthetic training data when real-world labeled datasets are scarce.
- Audio: Our models analyze speech waveform characteristics, spectrogram patterns, and linguistic inconsistencies to detect synthetic speech. By incorporating signal processing techniques, we ensure robustness against audio compression and noise interference.
- Videos: We detect falsified videos by tracking physiological inconsistencies in facial expressions, head movements, and blinking patterns. Our POI-specific detection models employ biometric representations to compare test videos against an enrolled dataset of genuine recordings, offering tailored protection against impersonation.
- Attribution: Tracing the source of manipulated media
Attribution helps determine who or what generated a piece of synthetic content, providing critical insights for identifying coordinated campaigns to deceive the public.- Text & Speech: We leverage stylometric analysis and deep learning to classify text by generator type, attributing it to specific language models. Similarly, our speech attribution models analyze voice synthesis artifacts to distinguish between text-to-speech and real-time voice cloning systems.
- Video: Our dataset of thousands of manipulated videos across multiple generation techniques provides the foundation for attribution research. We employ feature-space clustering and generative model fingerprinting to identify commonalities in synthetic video content.
- Images: Using image compression analysis and forensic watermarking detection, we validate the authenticity of news images. Our algorithms have been instrumental in uncovering high-profile media manipulation campaigns, such as the Russian Doppelganger project.
- Characterization: Understanding intent and impact
Not all manipulated content is malicious—some is satire, while others spread harmful propaganda. Our characterization techniques help distinguish intent and impact.- Context Awareness: We apply multimodal AI models that integrate textual, visual, and audio data to detect cross-modal inconsistencies. These techniques help identify recontextualized media, such as mislabeled images or AI-generated voices overlaid onto real video footage.
- Localization Tools: Our models pinpoint manipulated areas within media by generating heatmaps that highlight alterations in text, images, and videos. This fine-grained analysis helps analysts focus on critical regions of interest.
- Specialized Applications: We have developed targeted characterization techniques for high-profile topics, including automated agenda detection in news articles and social media sentiment analysis for media manipulation campaigns.
Advancing the Fight Against AI-Generated Content
Through the SemaFor program, Kitware has pushed the boundaries of media forensics, publishing nearly 50 peer-reviewed research papers and releasing benchmark datasets in detecting and analyzing manipulated media.
Our ongoing efforts include developing open-set characterization techniques to go beyond predefined intent categories and tackling AI-driven social engineering attacks, which represent the next wave of digital threats. As generative AI continues to evolve, so too must our strategies for defending against its misuse.
By advancing state-of-the-art forensic techniques, Kitware remains committed to safeguarding digital information and ensuring a more secure future.
Learn More
Interested in our research? Contact our team for more details on our work in media forensics and ethical AI.
Disclaimer: This research was developed with funding from the Defense Advanced Research Projects Agency (DARPA). The views, opinions and/or findings expressed are those of the author and should not be interpreted as representing the official views or policies of the Department of Defense or the U.S. Government.