A Framework for Challenge Design: Addressing Medical Computing Problems
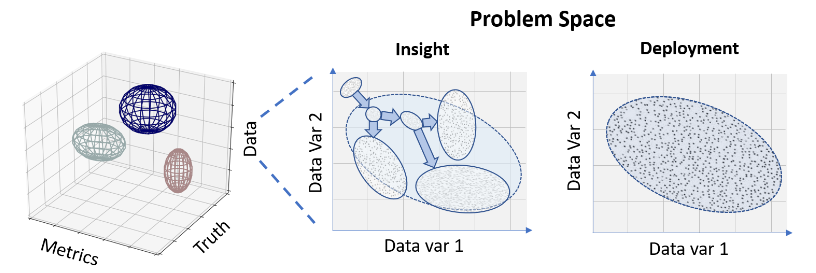
Need to determine a promising new research direction or assess the state of the art for your new product or process? Hosting a “challenge” may be an outstanding option; however, designing a challenge involves the careful consideration of both qualitative and quantitative experimental methods…and the popular cry for “more data” often becomes less critical when those methods are appropriately considered.
Challenges have a rich history in open science and medical computing. A challenge is an online competition that uses data, truth, and metrics to evaluate the performance of algorithms, submitted by participants, with respect to a research problem. In the medical image analysis field, close to 200 challenges have been organized thus far. They have, for example, fostered the widespread pursuit of mutual information for registration and deep learning for segmentation. Well designed challenges have the potential to focus the field on creating solutions to important problems as well as generating new insights and identifying common themes for future research. Poorly designed challenges, however, can equally impede a field, e.g. lead to false performance expectations and promote unfruitful research directions.
Led by Dr. Adriënne Mendrik (Netherlands eScience Center, The Netherlands), we have published a paper that presents a framework for challenge design that guides challenge organizers, participants, and observers through the challenge assessment process. Our challenge framework categorizes challenges as (1) insight challenges that are based on qualitative statistics and that can help identify fruitful research; and (2) deployment challenges that use quantitative statistics to identify solutions to specific problems. In this context, data sampling techniques must be considered before data sample size, notions of statistical significance are replaced by a focus on practical significance and generalization, and the importance of avoiding data saturation becomes clear. If these topics interest you, our paper is freely available on arXiv.
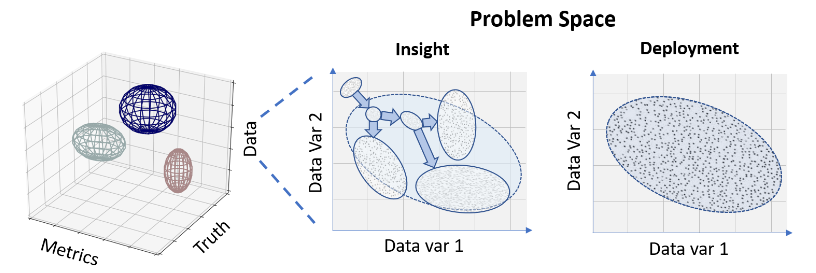