American Football Plays Paper Presented
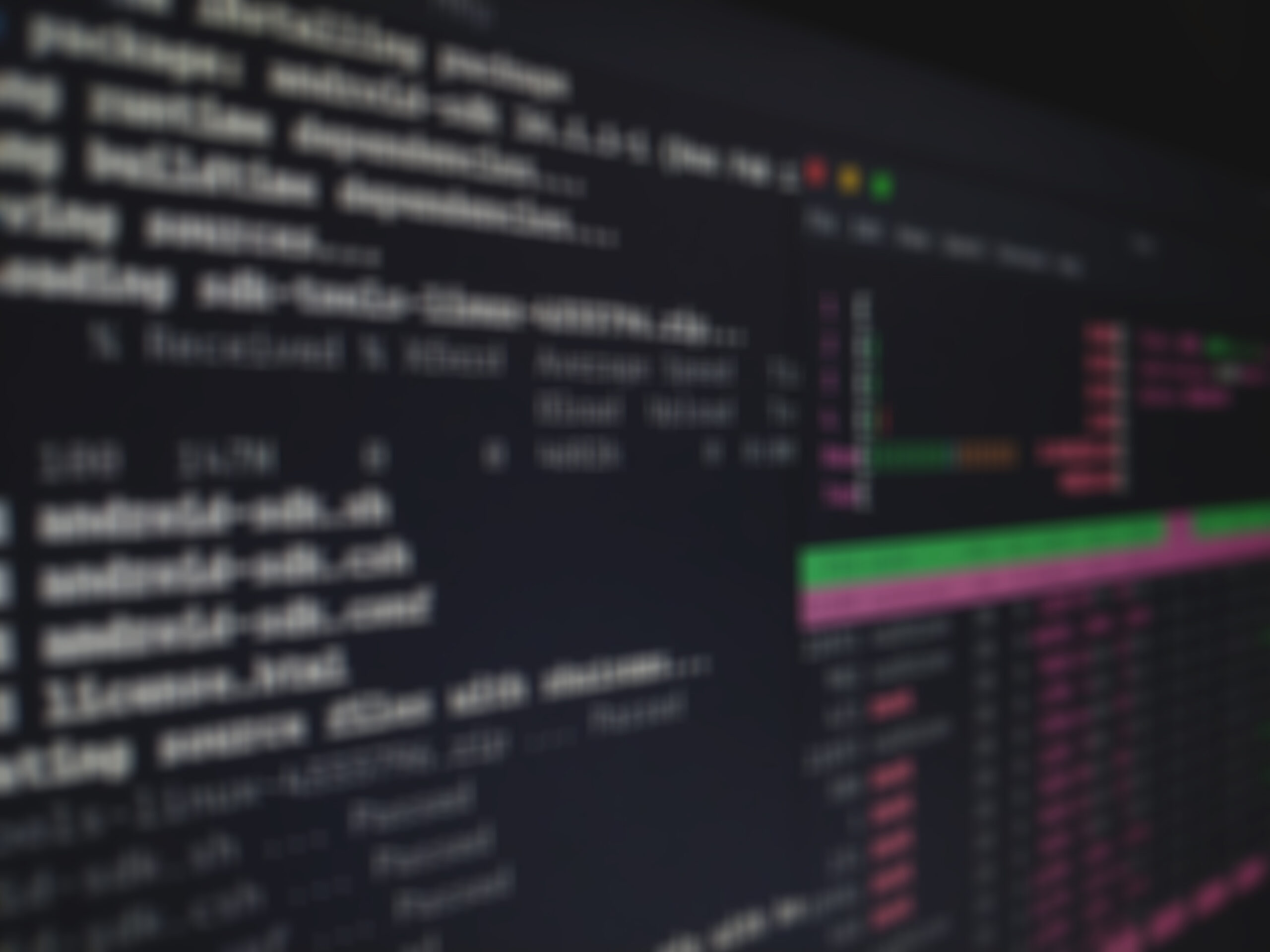
Eran Swears presented a paper at the WACV conference in Breckenridge Colorado on January 11 entitled: Learning and Recognizing Complex Multi-Agent Activities with Applications to American Football Plays. The paper, authored by Eran Swears and Anthony Hoogs, will be published in the Workshop IEEE Publication.
The basic research, which stems from the DARPA CARVE program, seeks to determine if we can automatically detect pre-planned or scripted coordinated activities using automatically computed and highly fragmented tracks from video. American football was chosen as a surrogate problem domain as it shares many of the difficulties of reconnaissance video, including difficult tracking, interacting agents (players), high variability within the same play type, and active deception.
The approach pushes the model complexity onto the observations by using a multi-variate kernel density while maintaining a simple HMM model. The temporal interactions of objects are captured by coupling the kernel observation distributions with a time-varying state-transition matrix, producing a Non-Stationary Kernel HMM (NSK-HMM). This modeling philosophy specifically addresses several issues that plague the more complex stationary models with simple observations, i.e. Dynamic Multi-Linked HMM (DML-HMM) and the Time-Delayed Probabilistic Graphical Model (TDPGM). These include: smaller training datasets, sensitivity to intra class variability and/or dense uninformative clutter tracks. Experiments are performed in the American football video domain, where the offensive plays are the activities. Comparisons are made to the DML-HMM and an extension of the TDPGM to DBNs (TDDBN). The NSK-HMM achieves a 57.7% classification accuracy across seven activities, while the DML-HMM is 26.7% and the TDDBN is 21.3%. When tested on four activities the NSK-HMM achieves a 76.0% accuracy.