Kitware Receives Honorable Mention for Explainable AI Toolkit
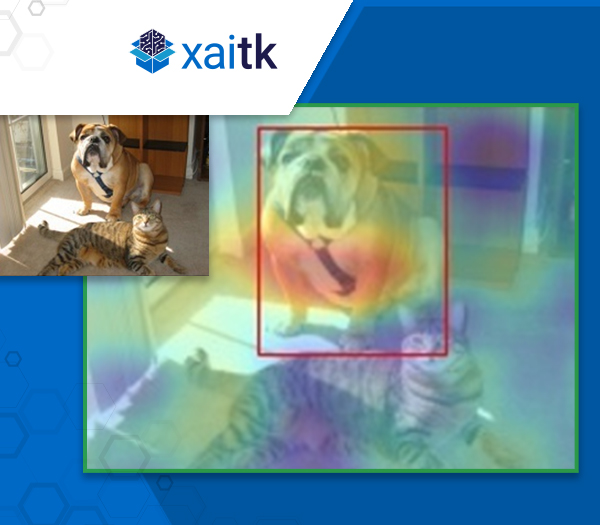
Through our long history and commitment to open source software development and our expertise in ethical and explainable AI, Kitware developed an open source tool called xaitk-saliency as part of a larger explainable AI toolkit. Xaitk-saliency provides a software framework for visual saliency algorithm interfaces and implementations so people can better understand how their AI models make predictions and decisions. To increase visibility around the toolkit, we submitted xaitk-saliency to Pytorch’s Annual Hackathon 2021 and are proud to have finished with an honorable mention in the responsible AI category. The toolkit is also now recognized by Facebook as being compatible with Pytorch, a major deep learning framework used by many AI researchers and professionals worldwide.
One of the main goals of xaitk-saliency is to create reproducible and sustainable software frameworks around critical explainable AI capabilities, with saliency maps being the form of visual explanation. This makes it valuable to those who are looking to incorporate explainability into their workflows. For example, xaitk-saliency is currently a centerpiece in our talks with the Joint AI Center (JAIC) about incorporating explainability in their testing and validation pipelines.
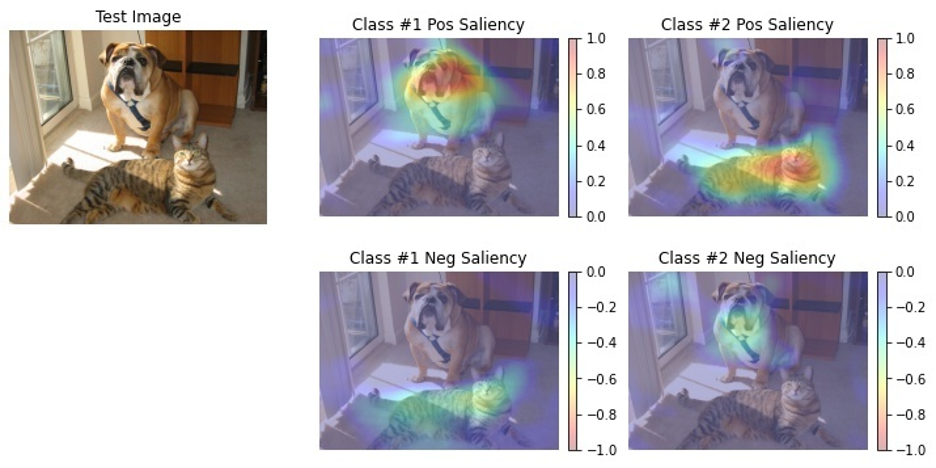
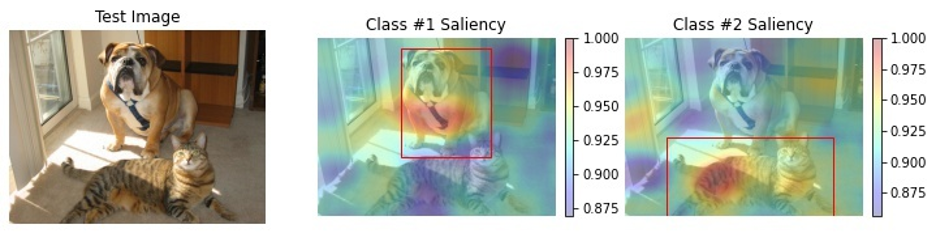
The xaitk-saliency package is part of Kitware’s larger open source Explainable AI Toolkit (XAITK), which was funded by DARPA as part of their Explainable AI program. XAITK contains a variety of tools and resources to help users, developers, and researchers understand and appropriately trust complex machine learning models. Our goals for XAITK are to consolidate and curate research results from the four-year DARPA XAI program into a single publicly accessible repository and to identify partnering opportunities with commercial and government organizations who want to implement XAI into their workflows. To learn more about XAITK, read our recently published, peer-reviewed paper.
This material is based upon work supported by the United States Air Force and DARPA under Cooperative Agreement numbers N66001-17-2-4028 and FA875021C0130. The views, opinions, and/or findings expressed are those of the author(s) and should not be interpreted as representing the official views or policies of the Department of Defense or the U.S. Government.