Video Analysis on Business Intelligence, Studies in Computational Intelligence
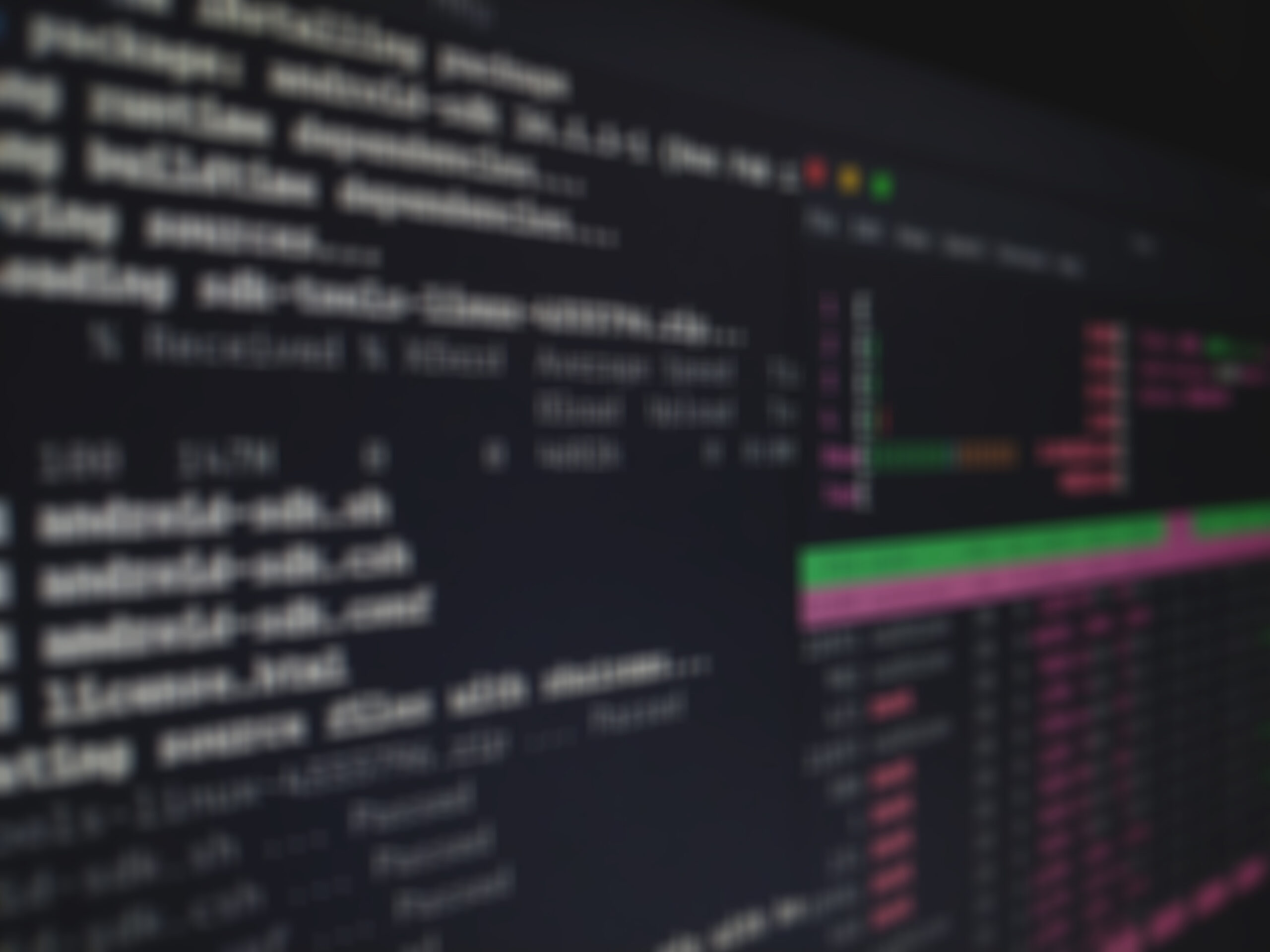
Over a year ago, Dr. Fatih Porikli, the Associate Editor for the Springer Journal on Machine Vision Applications and the Springer Journal on Real-time Image and Video Processing, approached Kitware Vision about submitting a book chapter proposal on “Video Analysis on Business Intelligence”. Dr. Fatih Porikli was looking for content relevant to the business intelligence aspects of video camera systems and was aware of Kitware’s expertise in the area of video analysis.
Although Kitware does not do much in the area of Business Intelligence, we came up with an idea related to the research we were doing on the Building Labeling in Urban Environments (BLUE) STTR DARPA sponsored program. BLUE’s goal is to combine movers and scene elements from video data to classify buildings by their surrounding activity as shown below.
Figure 1: Ocean City (OC) NJ video, where shops are mostly on the left side of the street.
Figure 2: Heat map showing all tracks for one day of OC webcam video; dark red indicates larger moving object bounding boxes while dark blue indicates smaller ones.
Figure 3: Likelihood distributions from each functional activity detector shown as heat maps; starting in the top left and going clockwise the functional elements are: doorway, walkway, roadway, and parking-spot; darker red indicates more likely regions, while darker blue is less likely.
Figure 4: Results of unsupervised functional element recognition; regions with the same color are the same functional category.
Our approach uses surveillance video to analyze normal pedestrian and vehicle behaviors around a set of store fronts in order to automatically analyze patterns that potentially impact a business. To accomplish this, we compared two functional recognition approaches and then integrated them into a more robust and accurate algorithm for improved analysis of business activities.
The first approach [1] uses supervised track-type classification for a person, driving-vehicle, parking-vehicle, and other classifications that are used as features in weak functional activity detectors to accumulate evidence for function element recognition; these include walkways, roadways, parking-spots, and doorways. The second approach [2] is a fully unsupervised method that identifies functional regions with similar behaviors by clustering histograms based on a trajectory level behavioral codebook. The likelihood maps from [1] are then incorporated into a clustering approach derived from [2] to produce more descriptive and consistent functional regions. The functional regions represent mixtures of functional elements, where one region can have a high concentration of a doorway element as well as a walkway element. Activity profiles can then be extracted from regions with a high concentration of the element of interest (doorway) in order to calculate business related statistics, i.e. number of pedestrians that pass by a store vs. enter/exit in a given scene.
The editors at Springer loved the proposed concept, and Kitware wrote the chapter entitled: “Automatic Activity Profile Generation from Detected Functional Regions for Video Scene Analysis,” with credits going to Eran Swears, Matthew Turek, Roderic Collins, Amitha Perera, and Anthony Hoogs. The book, titled “Video Analysis on Business Intelligence, Studies in Computational Intelligence,” will be published sometime this year by Springer, the leading global scientific publisher.
References
[1] E. Swears and A. Hoogs, “Functional Scene Element Recognition for Video Scene Analysis,” WMVC 2009
[2] M. Turek, A. Hoogs, R. Collins, “Unsupervised Learning of Functional Categories in Video Scenes,” ECCV2010
Eran Swears is an R&D Engineer on the Computer Vision team at Kitware. He leads the Phase 2 BLUE SBIR and has been the principle researcher on several other DARPA efforts. He specializes in event/activity modeling and recognition using graphical models and probabilistic logic with applications to computer vision.