Year in Review: Videos
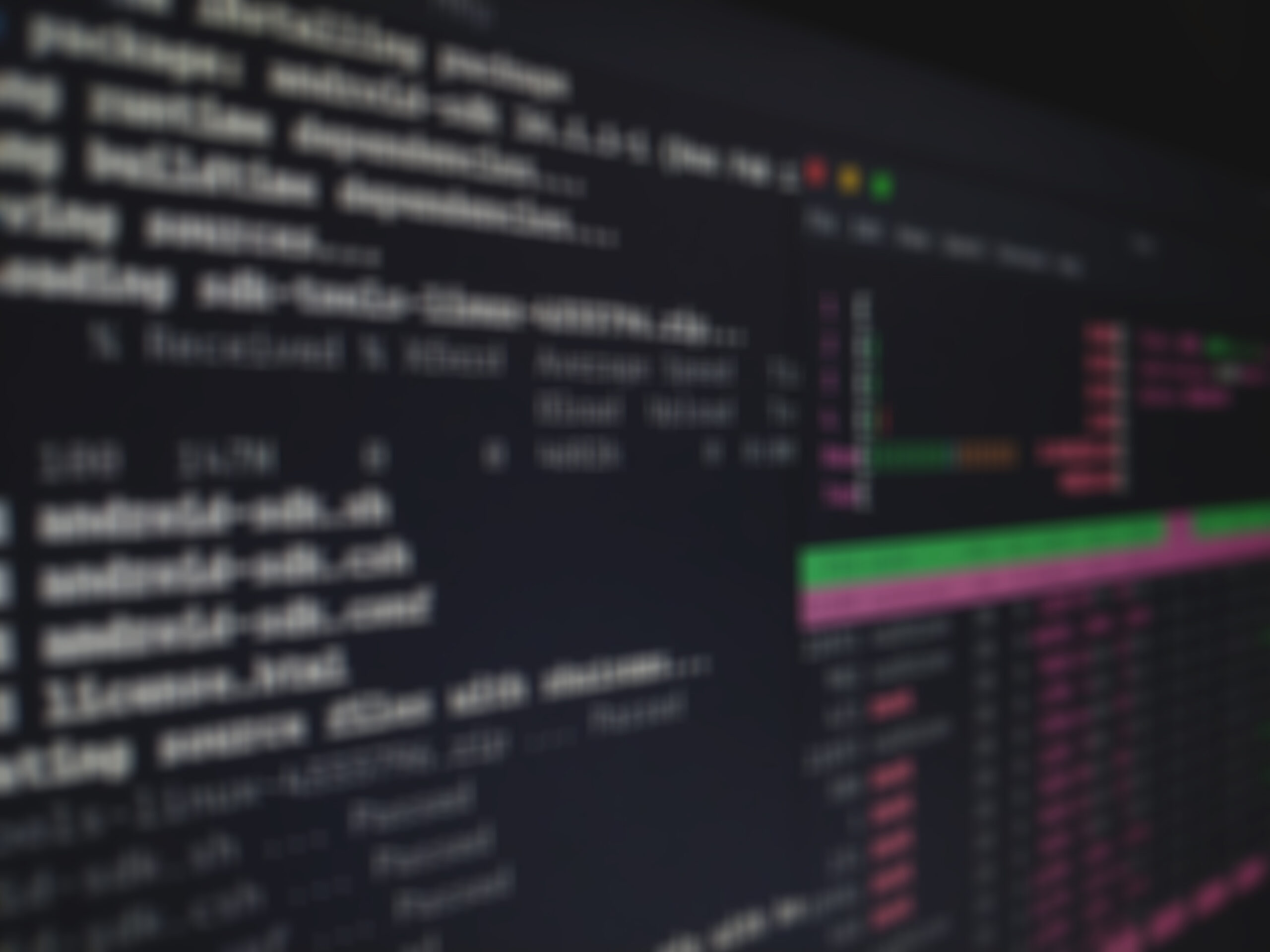
We had a busy year! Here are five of the many videos we made in 2014. To watch more of Kitware's videos, please see our Vimeo page.
1. Ebola Outbreak Timeline and Demonstration Video
We created a video that traces the outbreak from its onset in Guinea to other countries including Spain and the United States. The video depicts a sequence of key events that played a major role in spreading or introducing the Ebola disease to neighboring and distant nations. In this visual story of the 2014 Ebola Outbreak, the key events are organized on a horizontal timeline and are displayed in sequence at the appropriate time during the animation. The dataset was collected from various news reports, from WHO, and from other resources.
2. Fusing Surface Models and Medical Images, using the Structure Sensor and 3D Slicer
Registering the surface model of a patient with an MRI or CT scan of that patient is used in surgical planning, guidance, and simulation. Such registrations are common in high-end commercial medical systems; here we show how to perform them using the low-cost, innovative Structure Sensor and the open-source 3D Slicer application.
3. Interactive Visualization of Google Project Tango Data with ParaView
This video illustrates ParaView as a tool for visualizing and analyzing Google Project Tango data. ParaView is used to visualize and animate point clouds and sensor position, and VTK and PCL are used for example analysis pipelines.
4. SuperComputing 2014 Kitware
A compilation of videos from Kitware and our collaborators that we featured at our ParaView Showcase at SC14.
5. Complex Activity Recognition using Granger Constrained DBN (GDBN) in Sports and Surveillance Video
Kitware collaborated with RPI’s Professor Boyer, Eran Swears’ Ph.D. advisor, on a paper that was acceptetd at CVPR this year as a poster, which Eran presented. The poster is titled “Complex Activity Recognition using Granger Constrained DBN (GDBN) in Sports and Surveillance Video.” Here is the abstract from the paper:
Modeling interactions of multiple co-occurring objects in a complex activity is becoming increasingly popular in the video domain. The Dynamic Bayesian Network (DBN) has been applied to this problem in the past due to its natural ability to statistically capture complex temporal dependencies. However, standard DBN structure learning algorithms are generatively learned, require manual structure definitions, and/or are computationally complex or restrictive. We propose a novel structure learning solution that fuses the Granger Causality statistic, a direct measure of temporal dependence, with the Adaboost feature selection algorithm to automatically constrain the temporal links of a DBN in a discriminative manner. This approach enables us to completely define the DBN structure prior to parameter learning, which reduces computational complexity in addition to providing a more descriptive structure. We refer to this modeling approach as the Granger Constraints DBN (GCDBN). Our experiments show how the GCDBN outperforms two of the most relevant state-of-the-art graphical models in complex activity classification on handball video data, surveillance data, and synthetic data.