COMPUTER VISION AND PATTERN RECOGNITION CONFERENCE (CVPR)
Hosted by IEEE / CVF
June 18-22, 2023 at the Vancouver Convention Center in Vancouver, Canada
CVPR is the largest annual computer vision event, attracting students, academics, and industry researchers from around the world. CVPR covers a wide range of topics related to computer vision and machine learning, including object detection, image segmentation, 3D reconstruction, video analysis, and deep learning. Kitware has an extensive history of participating at CVPR as a leader in the computer vision community. Anthony Hoogs, Ph.D., vice president of artificial intelligence at Kitware, is serving as area chair for CVPR 2023.
Join one of the most recognized and credentialed providers of advanced computer vision research across the DoD and IC
Kitware’s Computer Vision Team is a leader in creating cutting-edge algorithms and software for automated image and video analysis, with more than two centuries of combined experience across our team in AI/ML and computer vision R&D. Our solutions have made a positive impact on government agencies, commercial organizations, and academic institutions worldwide.
Join our team to enjoy numerous benefits, including support to publish your novel work, the ability to attend national and international conferences, and more (you can see all of our benefits by visiting kitware.com/careers). If you’re interested in meeting with us to discuss our open positions, stop by our booth #1432.
Kitware’s Activities and Involvement at CVPR 2023
In addition to being an exhibitor, Kitware is a Silver sponsor of CVPR 2023. We are pleased to have the following paper in the main conference and are a sponsor of the EarthVision workshop.
Open Set Action Recognition via Multi-Label Evidential Learning
Thursday, June 22, from 4:30-6:30 PM | Poster Presentation #227
Authors: Chen Zhao, Dawei Du, Ph.D. (Kitware), Anthony Hoogs, Ph.D. (Kitware), Christopher Funk, Ph.D. (Kitware)
Existing methods for open set action recognition focus on novelty detection that assumes video clips show a single action, which is unrealistic in the real world. Our paper proposes a new method for open set action recognition and novelty detection via MUlti-Label Evidential learning (MULE). MULE goes beyond previous novel action detection methods by addressing the more general problems of single or multiple actors in the same scene with simultaneous action(s) by any actor. Our Beta Evidential Neural Network estimates multi-action uncertainty with Beta densities based on actor-context-object relation representations. An evidence debiasing constraint is added to the objective function for optimization to reduce the static bias of video representations, which can incorrectly correlate predictions and static cues. We develop a primal-dual average scheme update-based learning algorithm to optimize the proposed problem and provide corresponding theoretical analysis. Besides, uncertainty and belief-based novelty estimation mechanisms are formulated to detect novel actions. Extensive experiments on two real-world video datasets show that our proposed approach achieves promising performance in single/multi-actor, single/multi-action settings.
![Novelty detection examples of single/multiple actor(s) with single/multiple action(s) in video [16, 38], where an actor is identified as novel (yellow) rather than being from a known category (cyan) in inference. Existing works [4, 6] on open set action recognition focus on single actor associated with single action (bottom-left), while our method can handle different situations.](https://www.kitware.com/main/wp-content/uploads/2023/06/image2-1024x504.png)
EarthVision: Large Scale Computer Vision for Remote Sensing Imagery
Sunday, June 18, All day | Workshop Sponsor
Earth observation and remote sensing are growing areas of investigation where computer vision, machine learning, and signal/image processing meet. The goal is to provide large-scale, consistent information about the surface of the Earth by exploiting data collected by airborne and spaceborne sensors. This can be used for a broad range of tasks, including detection to registration, data mining, and multi-sensor, multi-resolution, multi-temporal, and multi-modality fusion and regression. This benefits numerous applications, such as location-based services, online mapping services, large-scale surveillance, 3D urban modeling, navigation systems, natural hazard forecast and response, climate change monitoring, virtual habitat modeling, food security, and more. The high volume of data associated with earth observation requires highly automated scene interpretation workflows. This workshop will explore topics related to this, including using super-resolution in the spectral and spatial domain, hyperspectral and multispectral image processing, and reconstruction and segmentation of optical and lidar 3D point clouds. Learn more.
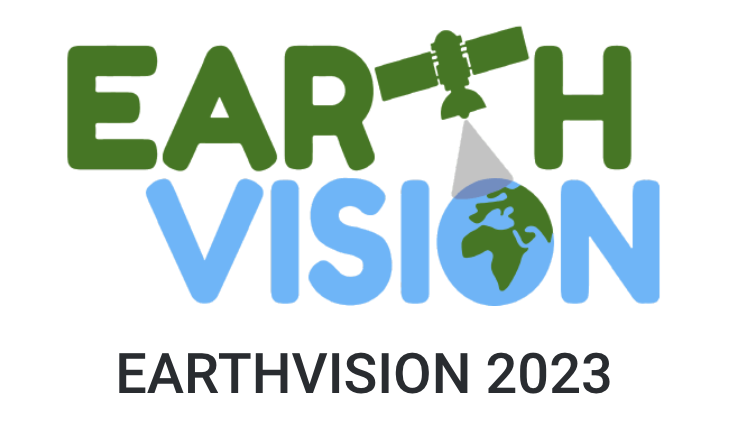
Kitware’s Computer Vision Work
Kitware is a leader in AI, machine learning, and computer vision. We have expertise in familiar areas, such as 3D reconstruction, complex activity detection, object detection and tracking, as well as emerging areas, such as explainable and ethical AI, interactive do-it-yourself AI, and cyber-physical systems. Kitware’s Computer Vision Team also develops robust lidar technology solutions for autonomy, driver assistance, mapping, and more. Our open source application, LidarView, performs real-time visualization and processing of live captured 3D lidar data. To learn more about Kitware’s computer vision work, you can email us at computervision@kitware.com.
Physical Event
Vancouver Convention Centre East Building
1055 Canada Pl, Vancouver, BC V6C 0C3, Canada