GEOINT 2020 Lightning Talk: Top 10 Tips and Tricks for Visual Data Labeling
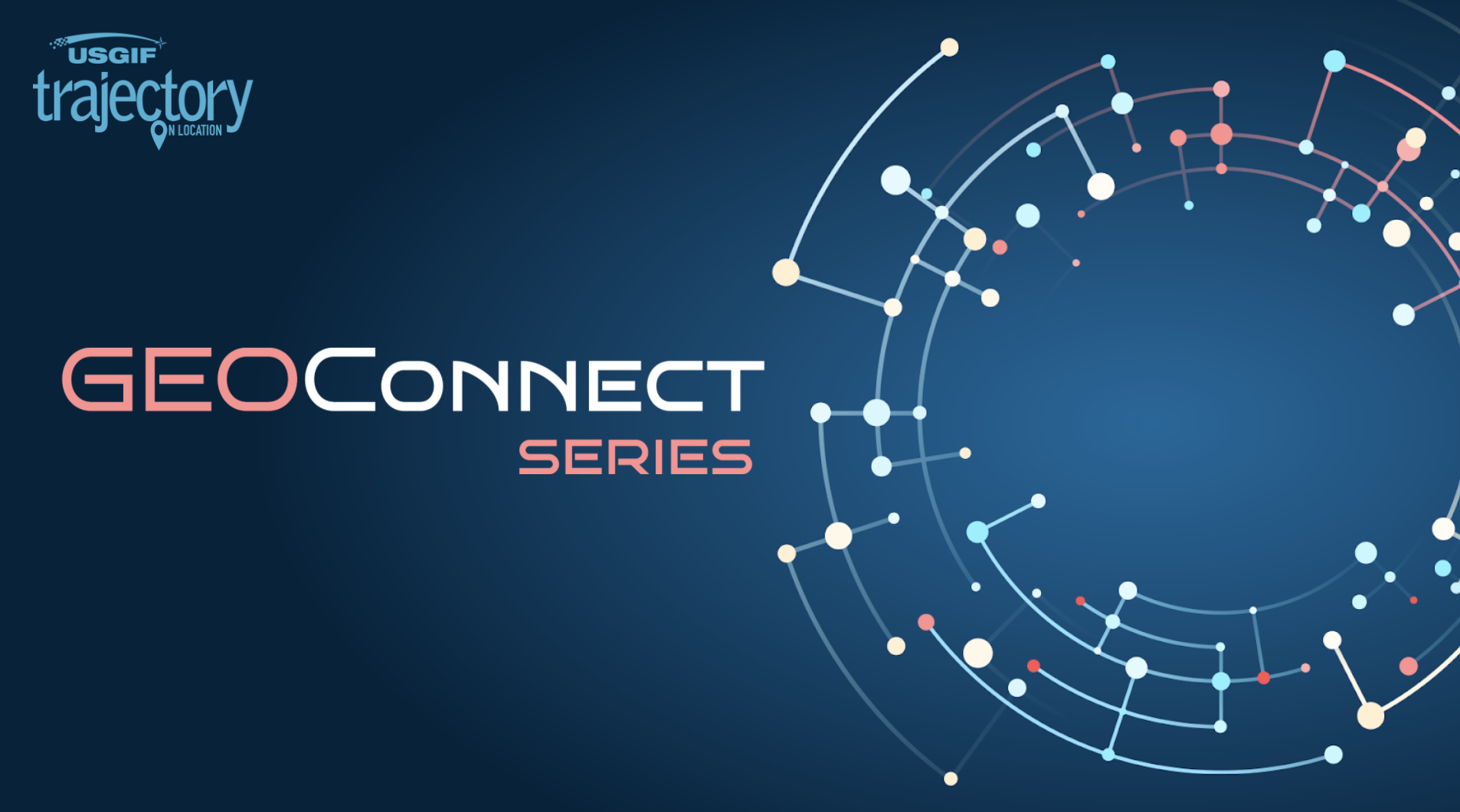
The GEOINT Symposium is held annually by the United States Geospatial Intelligence Foundation (USGIF). Though the event was canceled this year due to COVID-19, there are virtual education and networking opportunities still available, including GEOINT’s featured lightning talks. Kitware was thrilled to have its lighting talk proposal on visual data labeling accepted! As with all of the lightning talks this year, our presentation was recorded for online viewing and approved by the GEOINT lightning talk organizers. It will remain online indefinitely for viewing by anyone at no cost.
WATCH: Top 10 Tips and Tricks for Visual Data Labeling
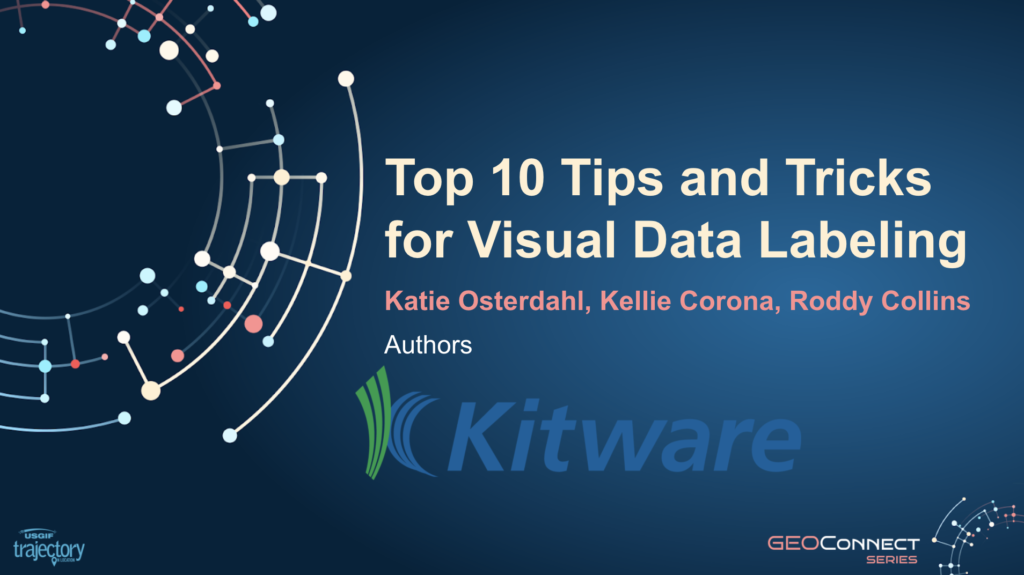
The increasing prevalence of using deep learning methods to solve GEOINT problems has resulted in high demand for labeled datasets to train these deep learning models. Since deep learning requires more annotated training data than traditional machine learning techniques, data labeling has become a bottleneck for organizations in terms of time, cost, and accuracy. Furthermore, designing, executing, and validating an effective data labeling process is surprisingly challenging, and many researchers, companies, and government labs have had to revise or even discard their labels after expending significant resources. Learning techniques to address these challenges is valuable to the GEOINT community.
This presentation covers lessons Kitware has learned from designing data collections and subsequently annotating the data. These include: designing the effort (data collection and annotation) to meet project goals and considering training versus evaluation needs; different types of pipelines for annotations and pros/cons of various labor pools; challenges of corner cases and how they need to be addressed depending on the type of dataset; and how these factors tie back into the cost of the overall effort.
Kitware’s Dataset Design and Annotation Expertise
Kitware’s computer vision team has been creating cutting-edge algorithms and software for automated image and video analysis since 2007. Our expertise includes visual dataset design, collection, curation, and annotation to meet our customer’s specific parameters.
One of our most recent efforts involved the creation of the Multiview Extended Video with Activities (MEVA) dataset, which was developed under the IARPA Deep Intermodal Video Analytics (DIVA) program. The MEVA dataset supports the DIVA performers and broader research community through activity detection in multi-camera environments. The publicly released data includes 328 hours of ground camera data and 4.6 hours of UAV data. We’ve released annotations for 22 hours of the ground camera data, and provide samples of the data and annotations on their respective websites.
In addition, Kitware created new annotations on the VIRAT Video Dataset as part of its work on the DIVA program. These annotations provide full tracks on all movers in the video data, with additional activity annotations for 46 activity classes. Kitware collaborated to create the VIRAT Video Dataset as part of the Defense Advanced Research Projects Agency (DARPA) Video and Image Retrieval and Analysis Tool (VIRAT) program. This dataset is designed to be realistic, natural and challenging for video surveillance domains in terms of its resolution, background clutter, diversity in scenes, and human activity/event categories than existing action recognition datasets.
Learn more about Kitware’s computer vision expertise and how it can be leveraged to benefit your research by visiting kitware.com/cv.
This research is based upon work supported in part by the Office of the Director of National Intelligence (ODNI), Intelligence Advanced Research Projects Activity (IARPA). The views and conclusions contained herein are those of the authors and should not be interpreted as necessarily representing the official policies, either expressed or implied, of ODNI, IARPA, or the U.S. Government. The U.S. Government is authorized to reproduce and distribute reprints for governmental purposes notwithstanding any copyright annotation therein.