GEOINT 2024
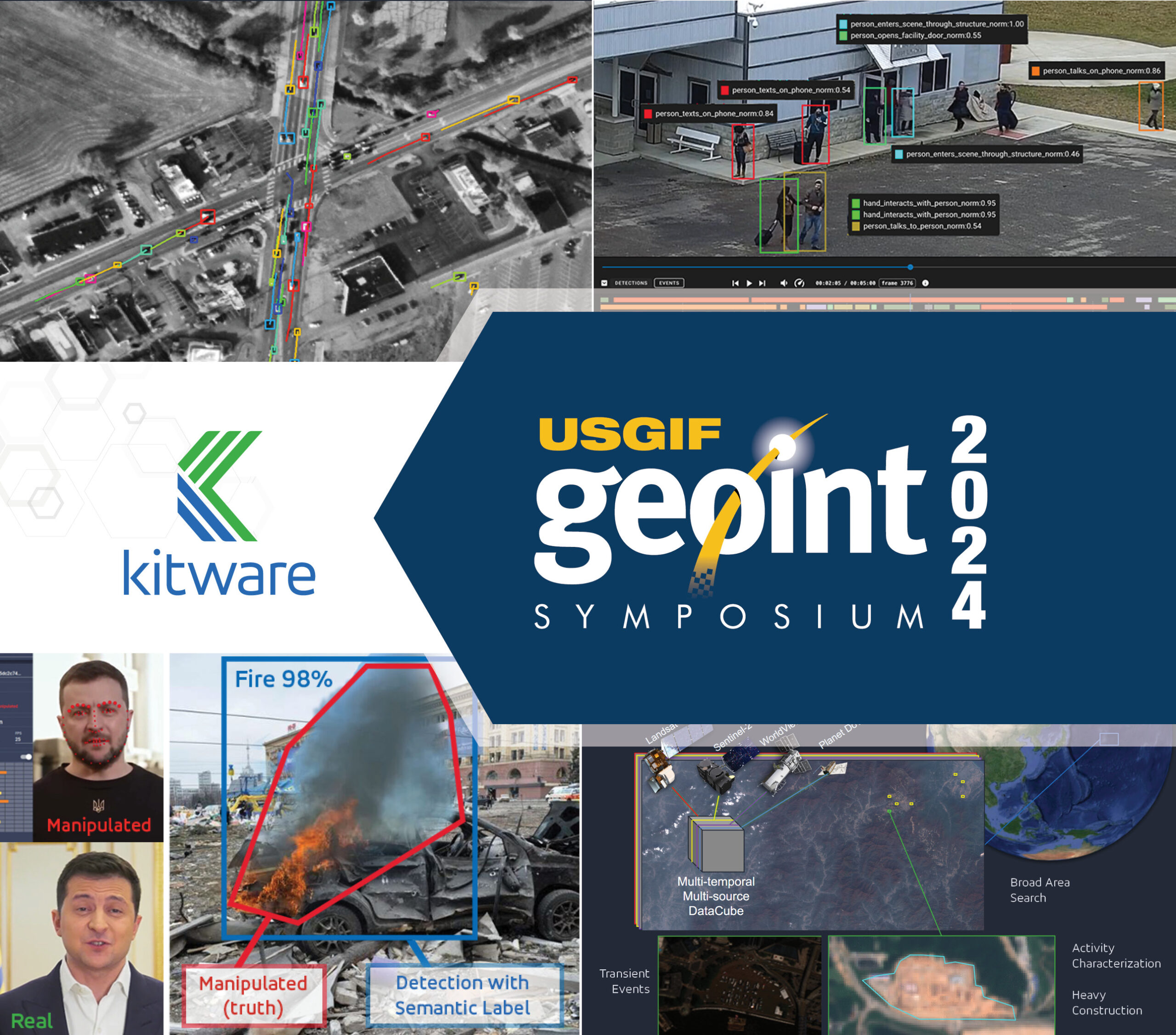
May 5-8, 2024 at the Gaylord Palms Resort and Conference Center in Kissimmee, Florida
The United States Geospatial Intelligence Foundation’s GEOINT Symposium is the largest gathering of geospatial intelligence professionals in the U.S., spanning across government, industry, and academia. Kitware has more than two centuries of combined experience in computer vision R&D for federal agencies. Our highly experienced and credentialed experts can help you solve the most challenging AI and computer vision problems in the GEOINT domain. Kitware is honored to be the most recognized and credentialed provider of advanced computer vision research across the DoD and IC, and we have showcased our work during the GEOINT training sessions and lightning talks for the past several years.
Kitware is excited to be involved again this year at GEOINT 2024 with three tutorial sessions and two lightning talks. In addition to these presentations, you can visit us at Booth 1719 to learn more about our geospatial AI capabilities and how we can help you solve difficult research problems. You can also schedule a meeting with our team.
Tutorials
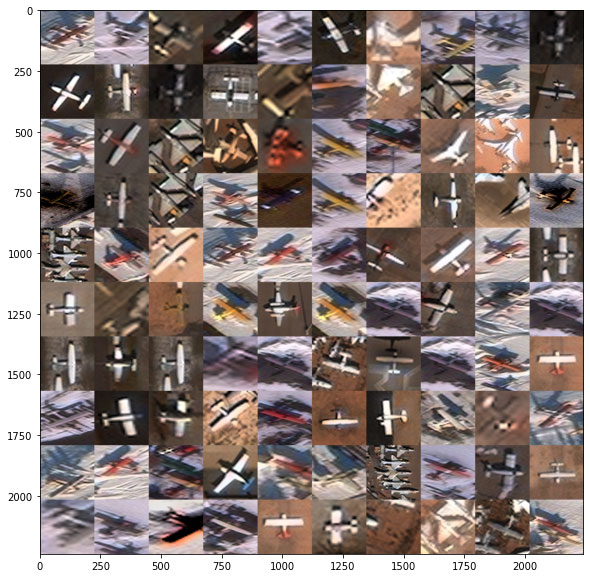
Adapting Large Language AI Models for GEOINT Applications
Presenter: Arslan Basharat, Ph.D
Monday, May 6 from 7:30-8:30 AM
Program Listing
ChatGPT and similar large language models have become popular for a variety of applications based on the broad swath of knowledge harvested from the internet. Adapting such models to the specific needs of a GEOINT analyst remains a challenge. As an example, we will use the domain of simulated medical triage as a specialized application where the pre-trained large language models have some but not a lot of background knowledge and expertise. We will leverage our work from the DARPA In the Moment (ITM) program to provide motivating examples, tools, and results from this domain. We will demonstrate how to adapt and refine some of the recent large language models with the text documents from the domain, e.g. TCCC medical triage guides. Furthermore, we will also demonstrate an approach to adapt the AI model’s decisions to be aligned with those of human decision-makers. Finally, we will also provide other potential adaptation examples that may be relevant to the GEOINT users.
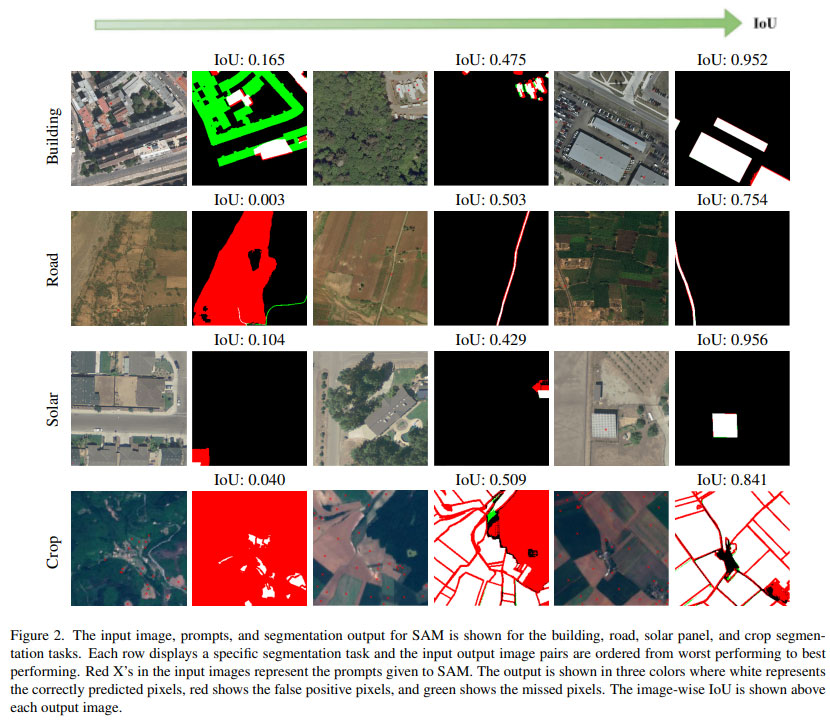
Language-Guided Vision for Remote Sensing
Presenter: Brian Clipp, Ph.D
Tuesday, May 7 from 7:30-8:30 AM
Program Listing
Recent advances in large, multimodal image and language models enable object detection, classification, segmentation, and tracking using only text prompts and imagery. Tasks that used to be trained with large-scale databases of labeled images can now be performed without any labeled in-domain data by leveraging large language-vision models pre-trained on massive foundational datasets. This training will introduce you to the latest research in language-guided data annotation, object detection, classification, segmentation, and tracking in remote sensing. We will emphasize functional demonstrations to highlight what works now. We will also introduce the transformer architecture and how it has revolutionized language and vision AI. This architecture has led to cross-trained, multi-modal systems that are enabling new, language-guided vision applications.
Using AI to Improve 3D Reconstruction from Remote Sensing Imagery
Presenter: Matt Leotta, Ph.D
Tuesday, May 7 from 2-3 PM
Program Listing
In the last few years, the methods used for reconstructing 3D geometry from images have undergone an AI revolution. These new methods are now being adapted to remote sensing applications as well. This training session will review recent developments in the computer vision and AI research communities as they pertain to 3D reconstruction from overhead imagery. We will provide a high-level summary of how traditional multi-view 3D reconstruction works, review the basics of neural networks, and then discuss how these fields have come together by reviewing and demystifying the recent research. In particular, we will discuss methods such as Neral Radiance Fields (NeRF) and Neural Implicit Surfaces for multiview 3D modeling. We will describe modifications and enhancements needed to adapt these methods from ground-level imagery to aerial and satellite imagery. We will also review recent work in using AI to estimate height maps from a single overhead image.
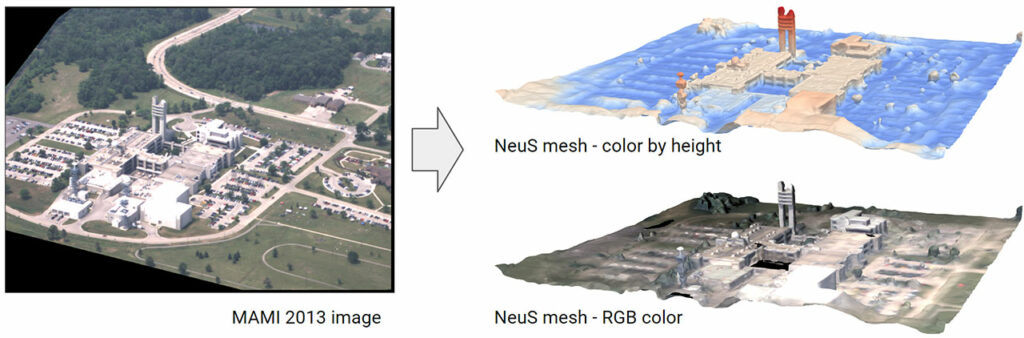
Lightning Talks
Hide and Seek at Megacity Scale
Presenter: Brian Clipp, Ph.D
Monday, May 6 from 3:30-3:35 PM
Program Listing
Currently, large-scale human movement modeling focuses on aggregated, high-level data to study migration, the spread of disease, and other patterns of behavior. For example, hourly toll booth counts indicate traffic volume. Kitware’s work on the IARPA HAYSTAC program aims to capture more fine-grained, individual human movement patterns to identify what characterizes “normal” movement while upholding public expectations for privacy. In contrast to toll booth counts, this could mean modeling the behavior of vehicles traveling along a toll road. Where do they originate from? Where do they typically go after the toll booth, and what stops do they make along a given route? How can we model this behavior without violating any individual’s privacy?
Toward these ends, Kitware is developing a privacy-preserving human movement simulation system designed to handle mega-cities of up to 30 million people and simulations for as long as an entire year. Our MIRROR simulator uses a parallel, multi-resolution, spatio-temporal decomposition approach to achieve state-of-the-art computational efficiency and scale. In our talk, we will describe the architecture of our simulator, including our novel combination of efficient mesoscopic, queue-based traffic simulation for traffic modeling and route generation with a parallel kinematic movement simulator that captures the detailed, meter-by-meter movement of vehicles.
In addition, we will describe approaches our team has developed to detect anomalous behaviors in trajectory data. These include more classical “feature engineering” based methods and novel transformer-based approaches. We will share initial anomaly detection results using our methods. Further, we will introduce methods we have developed to generate anomalous trajectories that meet certain goals, such as going to an unusual place or going to a usual place at an unusual time, but are hidden.
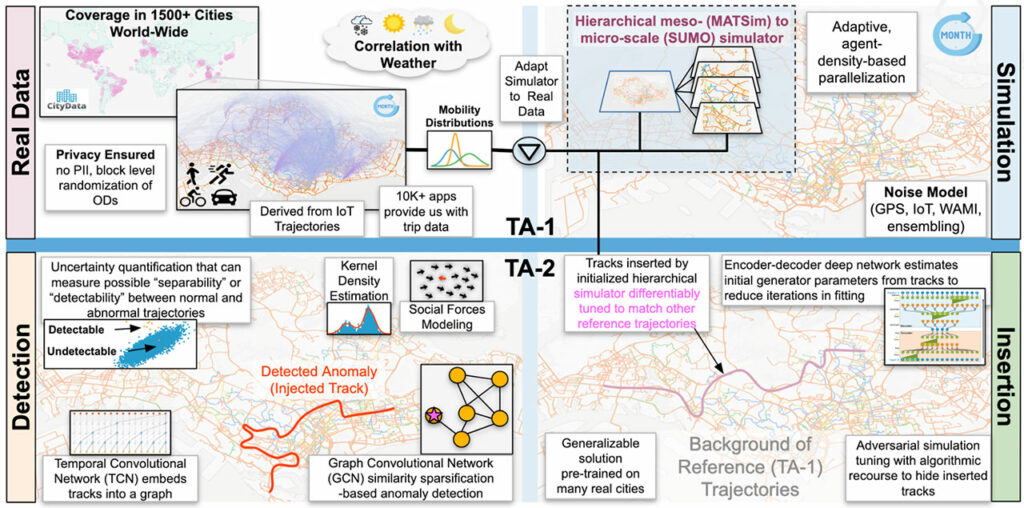
Updated Kitware Results on the IARPA SMART Program
Presenter: Matt Leotta, Ph.D
Tuesday, May 7 from 3:30-3:35 PM
Program Listing
The IARPA SMART program is bringing together many government agencies, companies, and universities to research new methods and build a system to search through enormous catalogs of satellite images from various sources to find and characterize relevant change events as they evolve over time. Imagery used includes Landsat, Sentinel-2, Worldview, and PlanetScope. Kitware is leading one of the performer teams on this program including DZYNE Technologies, Rutgers University, Washington University, and the University of Connecticut. Our team is addressing the problems of broad area search to find man-made change – initially construction sites. This is a “changing needle in a changing haystack” search effort. Our system further categorizes detected sites into stages of construction with defined geospatial and temporal bounds and will predict end dates for activities that are currently in progress. Our solution is called WATCH (Wide Area Terrestrial Change Hypercube) and has been deployed on AWS infrastructure to operate at scale. Furthermore, the software for WATCH, called “geowatch” has been released as open source and is freely available on Gitlab and PyPI.
In this talk, we will summarize significant progress made during the eighteen-month Phase 2 of the program, which ended in December, and show improvements since our previous results presented at GEOINT 2023. Notably, our detection scores on validation regions improved by a factor of three across Phase 2. We will briefly highlight our research results, system integration/deployment, and developed open source tools and AI models that are available for community use. We will conclude with a teaser of new directions in progress for Phase 3, including a new web-based user interface and tools to adapt the search to new tasks, such as the detection of short-term events (e.g., fairs and festivals).
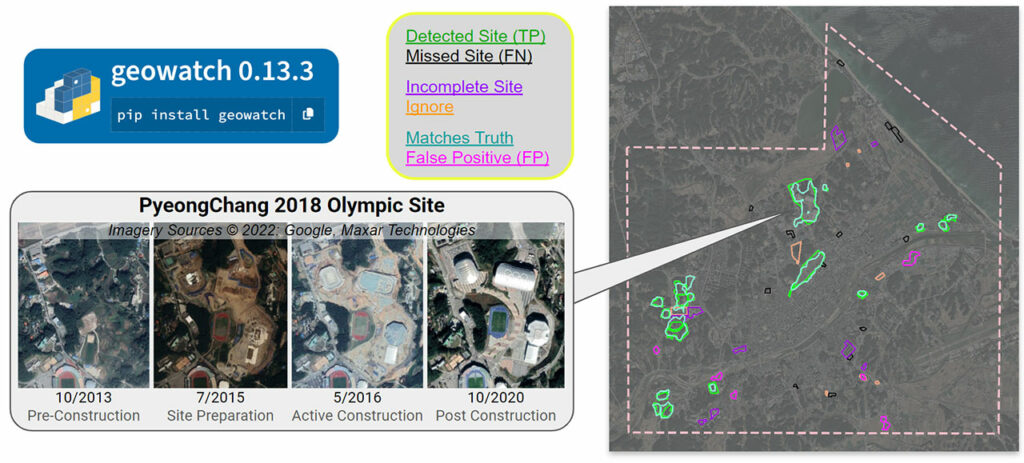
About Kitware’s Computer Vision Team
Kitware is a leader in AI/ML and computer vision. We use AI/ML to dramatically improve object detection and tracking, object recognition, scene understanding, and content-based retrieval. Our technical areas of focus include:
- Multimodal large language models
- Deep learning
- Dataset collection and annotation
- Interactive do-it-yourself AI
- Object detection, recognition, and tracking
- Explainable and ethical AI
- Cyber-physical systems
- Complex activity, event, and threat detection
- 3D reconstruction, point clouds, and odometry
- Disinformation detection
- Super-resolution and enhancement
- Computational imaging
- Semantic segmentation
- We also continuously explore and participate in other research and development areas for our customers as needed.
Kitware’s Computer Vision Team recognizes the value of leveraging our advanced computer vision and deep learning capabilities to support academia, industry, and the DoD and intelligence communities. We work with various government agencies, such as the Defense Advanced Research Project Agency (DARPA), Air Force Research Laboratory (AFRL), the Office of Naval Research (ONR), Intelligence Advanced Research Projects Activity (IARPA), and the U.S. Air Force. We also partner with many academic institutions, such as the University of California at Berkeley, Columbia, Notre Dame, the University of Pennsylvania, Rutgers, the University of Maryland at College Park, and the University of Illinois, on government contracts.
To learn more about Kitware’s computer vision work, check out our website or contact our team. We look forward to engaging with the GEOINT community and sharing information about Kitware’s ongoing research and development in AI/ML and computer vision as it relates to geospatial intelligence.